Unraveling Financial Behaviors (3): Digital Financial Service's Role as a Hub for Users’ Social Networking and Learning in Optimizing Remittance Timing
- Hanna Yim
- Feb 13, 2024
- 5 min read
Updated: Feb 16, 2024
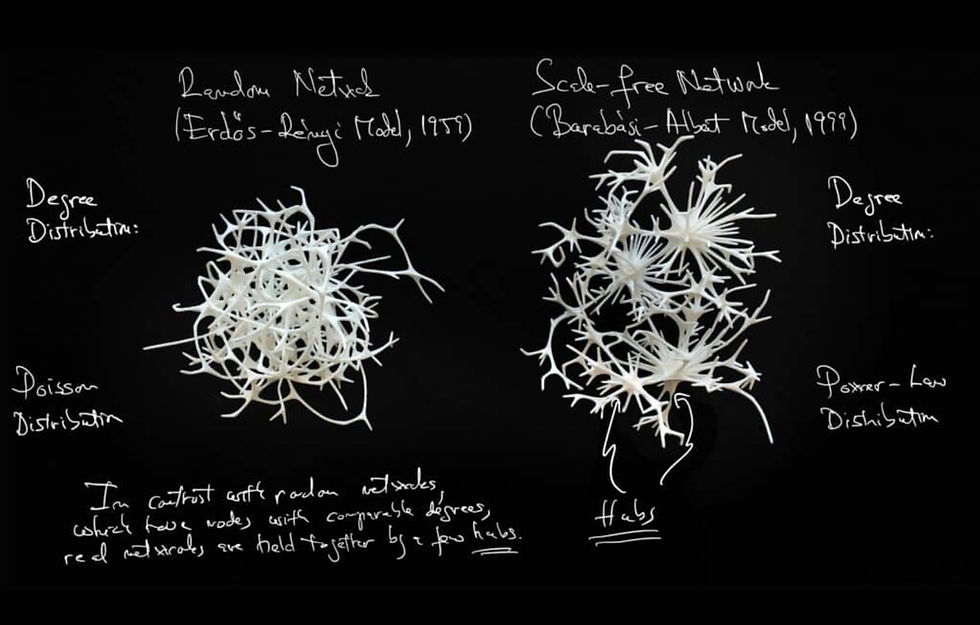
In our preceding blog posts (Unraveling Financial Behaviors (1), (2)), we delved into intriguing revelations surrounding SentBe's migrant worker users. We uncovered their remarkable sensitivity to exchange rate fluctuations when determining the timing of remittances, as well as their proactive use of the cancellation feature to capitalize on optimal exchange rates. Interestingly, a significant portion of our users, approximately 45%, have utilized this cancellation feature at least once, with 20% leveraging it multiple times to refine their remittance strategies. Surprisingly, SentBe had not actively promoted this feature, yet users seem to have organically discovered its advantages through their own exploration or by learning from fellow users. This narrative prompted us to explore further into the factors driving user adoption and learning regarding this feature.

SentBe's transaction dataset includes referral codes attributed to each user, akin to many online services across diverse sectors. Through SentBe's online remittance service referral program, existing users are incentivized to refer new users, thereby creating a network effect. When a new user signs up using a referral code, both the referrer and the new user enjoy waived remittance fees for their transactions. This referral data provides invaluable insights into user networks and their financial behaviors, particularly regarding exchange rate sensitivity and optimization strategies.
Analyzing this dataset offers a glimpse into the formation of user networks. For instance, Table 1 illustrates the sign-up journeys of 21 Filipino users, denoted alphabetically from A to U. Notably, User A introduced SentBe to 16 users, some - Users C, R, Q, and G - of whom further expanded the network by referring additional users. These interconnected clusters of users exhibit similar characteristics, including residential locations and occupations. Further scrutiny of the dataset revealed over 190 social networks, comprising approximately 10% of the total 14,078 sample users. Figure 1 visualizes one such social network, highlighting the referral relationships and interconnectedness among users.
Table 1. List of Users in a Social Network
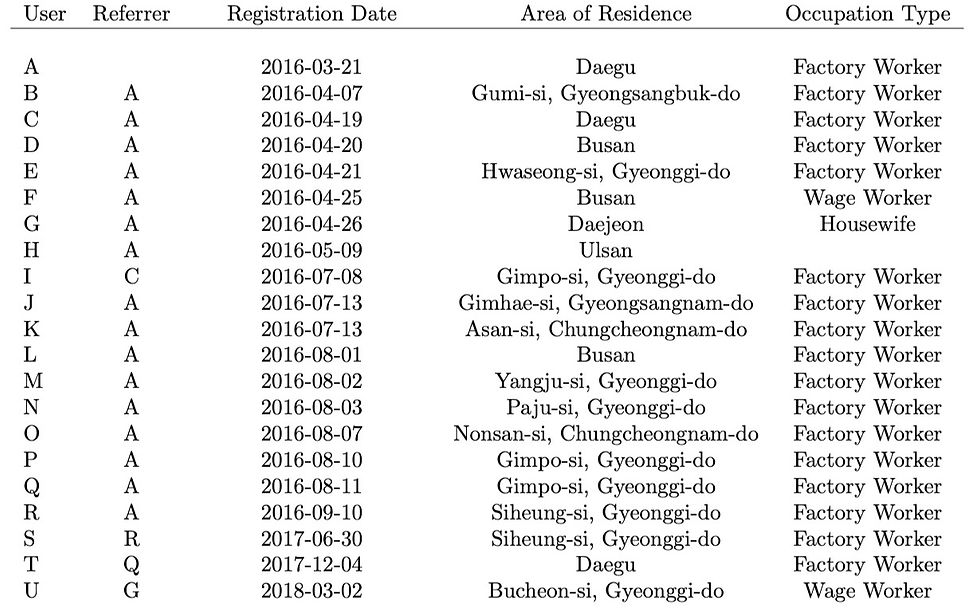
Source: Agarwal, S., Cho, S., Choi, H.-S., & Klapper, L. (2021). "Optimizing the Use of Fintech for International Remittances by Migrant Workers." KAIST College of Business Working Paper Series. Link
Figure 1. An Example of Social Network

Source: Ibid.
User transactions within these social networks exhibit clustering patterns, particularly in terms of remittance timing, as depicted in Table 2. Transactions among users within the same network tend to occur sequentially, suggesting a collaborative decision-making process influenced by network dynamics.
Table 2. Clustered Remittances Transactions
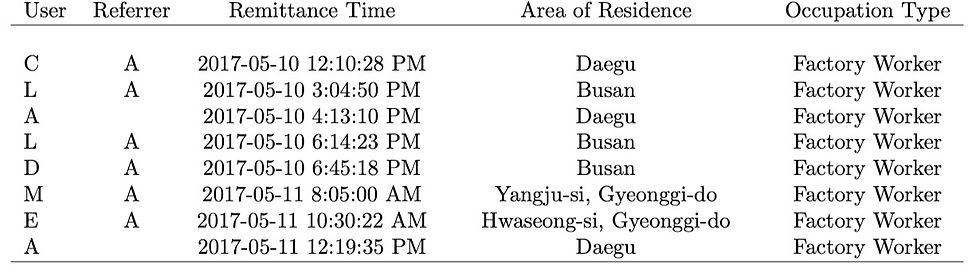
Source: Ibid.
This herding behavior becomes evident when compared to a control group (orange bar) of individual users not affiliated with social networks on SentBe, as illustrated in Figure 2. Users within social networks (blue bar) display more concentrated remittance transactions, indicating a collective influence on timing decisions.
Figure 2. An Example of Clustered Remittance Transactions in a Social Network
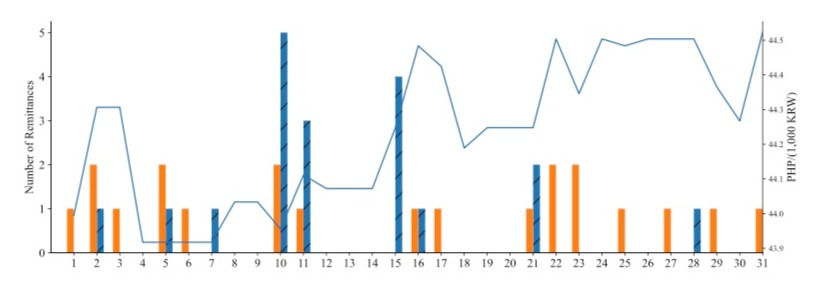
Source: Ibid.
The most fascinating aspect of our analysis lies in exploring how users within social networks on SentBe optimize their money transfers by leveraging practical insights into exchange rates. As highlighted in our earlier post, users often cancel remittance orders multiple times within a short period to capitalize on favorable exchange rates. Figure 3 provides a series of snapshots illustrating how the utilization of the sequential cancellation feature spreads throughout the expanding web of a social network. Each node in the network represents an individual user, with arrows indicating referral relationships. Users' nodes transition to grey once their usage of sequential cancellation is detected, with the size of the node increasing with each use.
To illustrate, consider a user who signed up on March 21st. By April 20th, three additional users had signed up through this initial user, yet none had utilized the cancellation feature. However, by July 8th, five more users joined the social network, with one user initiating the use of the cancellation option. Over time, starting from July 13th, the number of users with experience in cancelling remittance orders gradually increases, as evidenced by the growing number of grey-colored nodes in the network diagram. This phenomenon reflects the diffusion of information on the cancellation feature, facilitating collective learning among users within their social network.
Figure 3. An Example of Learning on Sequential Cancellation within a Social Network

Source: Ibid.
Does this collective learning translate into more optimal exchange rate decisions? The Optimality Score (OS) offers valuable insights into this question. One of the previous posts revealed that the OS of the cancellation group was 0.062, while it was 0.015 for those who had never used the cancellation option. However, in cases where users have used the cancellation function sequentially multiple times, the OS is shown to be at a much higher level, at 0.162. Further analysis demonstrates that users within social networks exhibit significantly higher OS values compared to independent users, particularly those leveraging sequential cancellations, as shown in Table 3. This suggests that the more actively users engage with the cancellation feature, especially in a social network context, the higher the level of optimality in the exchange rates applied to their transactions.
Table 3. Learning of Sequential Cancellation and Optimal Remittance Timing

Source: Ibid.
Further analysis delved deeper into the group of users utilizing the cancellation feature within social networks. SentBe users typically specify a transfer purpose when sending money. Leveraging this data, the study divided users into two sub-groups based on transfer purpose: "cost-of-living for family" (Living) and "other different reasons" (Others). Additionally, users were classified by income level, estimated by the average monthly transfer amount, into two sub-groups: Low and High. Examining the social learning of sequential cancellation within these sub-categories, users in the Living and Low income groups exhibited statistically significant social learning of sequential cancellation. The OS values for these groups were 1.101 and 1.176, respectively, whereas the Others and High income groups even ended up with negative OS values.
Table 4. Social Learning of Sequential Cancellation in Various Subgroups

Source: Ibid.
Researchers also explored the influence of the opening of SentBe’s offline customer service centers on the dissemination of information regarding the use of sequential cancellation. They examined a sample of users, referred to as "Treated," selected based on their registered residential address within a 50-kilometer radius of the city where SentBe’s customer service center is located. If a Treated user initiated at least one transaction through SentBe after the introduction of the offline center, they were classified into the "Post" group. Approximately 18% of the total sample comprised Treated senders. According to Table 5, the social learning of sequential cancellation was significantly intensified only among users associated with SentBe's social network who were part of the Treated and Post groups, demonstrating the notable impact of offline centers on user behavior.
Table 5. Offline Consumer Service Center Opening and Social Learning of Sequential Cancellation

Source: Ibid.
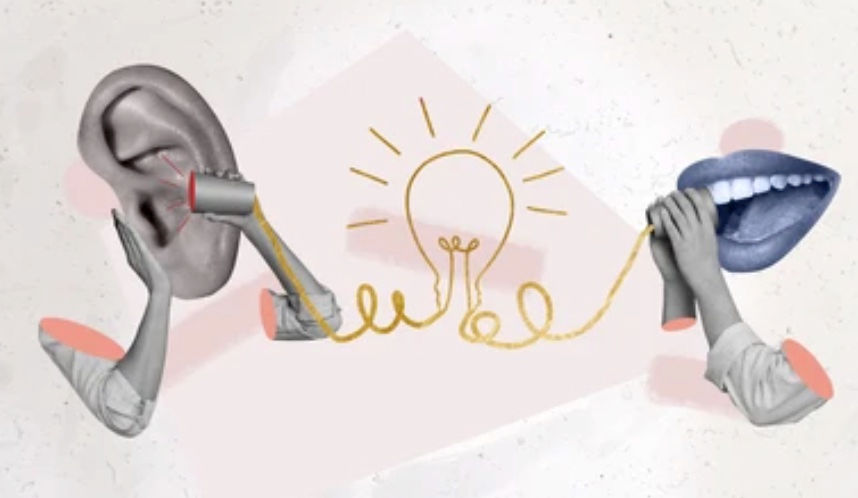
In concluding our exploration, SentBe emerges not only as a conduit for remittance transactions but also as a facilitator of social networking and collective learning. Our analysis has unveiled a multitude of insights into how users leverage the platform to optimize their remittance strategies. The collaborative decision-making observed within social networks, along with the dissemination of practical knowledge, underscores SentBe's role in empowering users and fostering financial inclusion within migrant communities.
コメント